报告题目:On Density-based Local Community Search
报告时间:2024年03月26日14:00
报告地点:bet356亚洲版体育官网B404会议室
报告人:乔淼
报告人国籍:中国
报告人单位:奥克兰大学
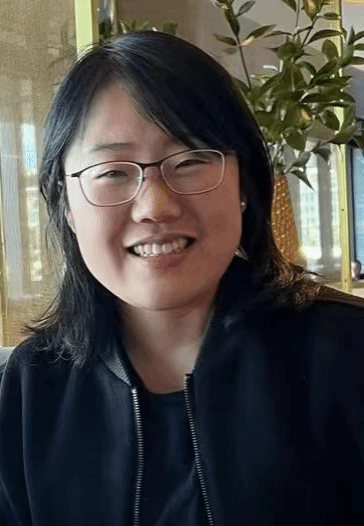
报告人简介:Miao Qiao received her PhD from the Chinese University of Hong Kong in 2013 and joined the School of Computer Science, the University of Auckland, in 2018. Her expertise lies in the theory and practice of databases. Her past and current research topics include indexing, query optimization, big data management and graph analytics. (1) Indexing and query optimization. Her past and ongoing research span over topics including graph distance queries, nearest neighbor search in high dimensional space, range thresholding queries on data streams, multi-way join queries in relational databases, local dense subgraph search, etc.(2) Efficient processing and analysis of big data, in particular graph data. Her past and ongoing research have explored the computation of different graph metrics, densest subgraph search, community detection, hypergraph clustering, I/O-efficient algorithm design and streaming algorithms.(3) Brain network analysis. This is a long-term and interdisciplinary (with medical and health science) research aiming at understanding and explaining the functions of the human brain, and bettering the predictions of the malfunctioning of the human brain with graph analytical techniques.Her research has been supported by Royal Society of New Zealand and the Ministry of Business, Innovation and Employment, New Zealand.
报告摘要: Given a graph G and a set R of seed nodes, local community search (LCS) reports a community that is local to R. Specifically, for an induced subgraph S of G, the objective function f(S) not only considers classic community measurement of S such as conductance and density, but also encodes set inclusion criteria of R; LCS optimizes f(S) over all the induced subgraphs of G. Ideally, the optimization algorithm for f(S) should be strongly local, that is, its complexity dependents on R as opposed to the entire graph G. We formulate a general form of objective functions for LCS using configurations --- one configuration corresponds to one LCS objective function. For the set C of configurations corresponding to density-based LCS, we i) find C_L \subseteq C in a constructive classification of C: a configuration in C has a strongly local algorithm for optimizing its corresponding objective function if and only if it is in C_L, and ii) provide a linear programming-based general solution for density-based LCS --- the solution is strongly local and ready to be deployed to practical scenarios.
邀请人:祝园园