学术报告(一)
报告题目:Neural Spectrospatial Filter: On Beamforming in the Deep Learning Era
报告时间:2023年7月10日(周一)上午9:30
报告地点:bet356亚洲版体育官网B404会议室
报告人:汪德亮
报告人国籍:美国
报告人单位:美国俄亥俄州立大学
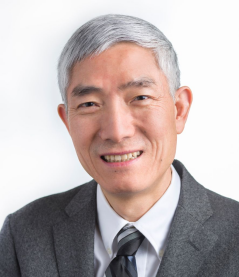
报告人简介:DeLiang Wang received the B.S. degree and the M.S. degree from Peking (Beijing) University and the Ph.D. degree in 1991 from the University of Southern California all in computer science. Since 1991, he has been with the Department of Computer Science & Engineering and the Center for Cognitive and Brain Sciences at The Ohio State University, where he is a Professor and University Distinguished Scholar. He received the U.S. Office of Naval Research Young Investigator Award in 1996, the 2008 Helmholtz Award from the International Neural Network Society, the 2007 Outstanding Paper Award of the IEEE Computational Intelligence Society and the 2019 Best Paper Award of the IEEE Signal Processing Society. He is an IEEE Fellow and ISCA Fellow, and currently serves as Co-Editor-in-Chief of Neural Networks.
报告摘要:As the most widely-used spatial filtering approach for multi-channel signal separation, beamforming extracts the target signal arriving from a specific direction. We present an emerging approach based on multi-channel complex spectral mapping, which trains a deep neural network (DNN) to directly estimate the real and imaginary spectrograms of the target signal from those of the multi-channel noisy mixture. In this all-neural approach, the trained DNN itself becomes a nonlinear, time-varying spectrospatial filter. How does this conceptually simple approach perform relative to commonly-used beamforming techniques on different array configurations and in different acoustic environments? We examine this issue systematically on speech dereverberation, speech enhancement, and speaker separation tasks. Comprehensive evaluations show that multi-channel complex spectral mapping achieves speech separation performance comparable to or better than beamforming for different array geometries, and reduces to monaural complex spectral mapping in single-channel conditions, demonstrating the versatility of this new approach for multi-channel and single-channel speech separation. In addition, such an approach is computationally more efficient than popular mask-based beamforming. We conclude that this neural spectrospatial filter is capable of superseding traditional and mask-based beamforming.
邀请人:杜博、涂卫平
学术报告(二)
报告题目:网络环境下的鲁棒语音处理与安全
报告时间:2023年7月10日(周一)上午10:30
报告地点:bet356亚洲版体育官网B404会议室
报告人:张晓雷
报告人国籍:中国
报告人单位:西北工业大学
报告人简介:张晓雷,西北工业大学教授,博导。清华大学博士、美国俄亥俄州立大学博士后。从事语音处理、机器学习、人工智能的研究工作。在Neural Networks、IEEE TPAMI、IEEE TASLP、IEEE TCYB、Computer Speech and Language等期刊、会议发表论文80余篇。出版专著1部、译著1部。承担国家重点研发计划、国家自然科学基金重点项目等省部级以上项目10余项。入选国家与省部级青年人才计划。获得国际神经网络学会最佳论文、亚太信号与信息处理学会杰出讲者、北京市科学技术一等奖等。目前担任Neural Networks、IEEE TASLP等国际期刊的编委、IEEE SLTC委员等。
报告摘要: 近年来,尽管大数据+深度学习在语音识别等任务上取得了显著突破,但是语音处理在远场、强自然环境噪声干扰和人造恶意攻击下仍然表现出了一定的脆弱性,限制了其在智慧城市等更大范围的应用。如何充分利用网络进行多设备安全互联是解决该问题的潜在方法之一。本报告将分享我们在该方向的一点探索,重点介绍自然噪声和远场环境下的分布式自组织阵列及智能语音应用技术、及声纹识别的对抗样本攻击与防御技术。
邀请人:杜博、涂卫平